M. Sc. Alice Moallemy-Oureh
Graphs in Artificial Intelligence and Neural Networks (GAIN)
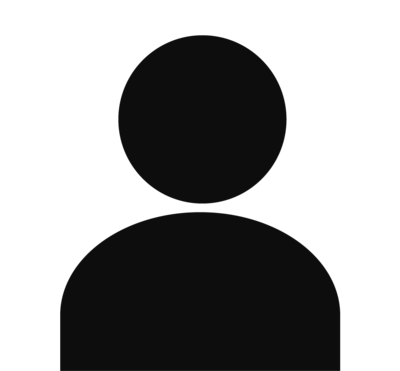
- Telephone
- +49 561 804-6363
- amoallemy[at]uni-kassel[dot]de
Publications
2024[ to top ]
- Weisfeiler–Lehman goes dynamic: An analysis of the expressive power of Graph Neural Networks for attributed and dynamic graphs. . In Neural Networks, 173, bl 106213. Elsevier, 2024.
2023[ to top ]
- Marked Neural Spatio-Temporal Point Process Involving a Dynamic Graph Neural Network. . In Workshop on Temporal Graph Learning (TGL), NeurIPS, bll 1–7. 2023.
- Graph Pooling Provably Improves Expressivity. . In Workshop on New Frontiers in Graph Learning, NeurIPS, bll 1–7. 2023.
- Graph Neural Networks Designed for Different Graph Types: A Survey. . In Transactions on Machine Learning Research. 2023.
2022[ to top ]
- Weisfeiler-Lehman goes Dynamic: An Analysis of the Expressive Power of Graph Neural Networks for Attributed and Dynamic Graphs. . In arXiv e-prints, bl arXiv:2210.03990. 2022.
- Student Research Abstract: Continuous-Time Generative Graph Neural Network for Attributed Dynamic Graphs. . In ACM/SIGAPP Symposium on Applied Computing (SAC), bll 600–603. ACM, 2022.
- On the Extension of the Weisfeiler-Lehman Hierarchy by WL Tests for Arbitrary Graphs. . In Workshp on Mining and Learning on Graphs (MLG), ECML PKDD, bll 1–13. 2022.
- Graph Neural Networks Designed for Different Graph Types: A Survey. . In arXiv e-prints, bl arXiv:2204.03080. 2022.
- FDGNN: Fully Dynamic Graph Neural Network. . In arXiv e-prints, bl arXiv:2206.03469. 2022.
2021[ to top ]
- A Note on the Modeling Power of Different Graph Types. . In arXiv e-prints, bl arXiv:2109.10708. 2021.